4 ways generative AI can solve manufacturing challenges
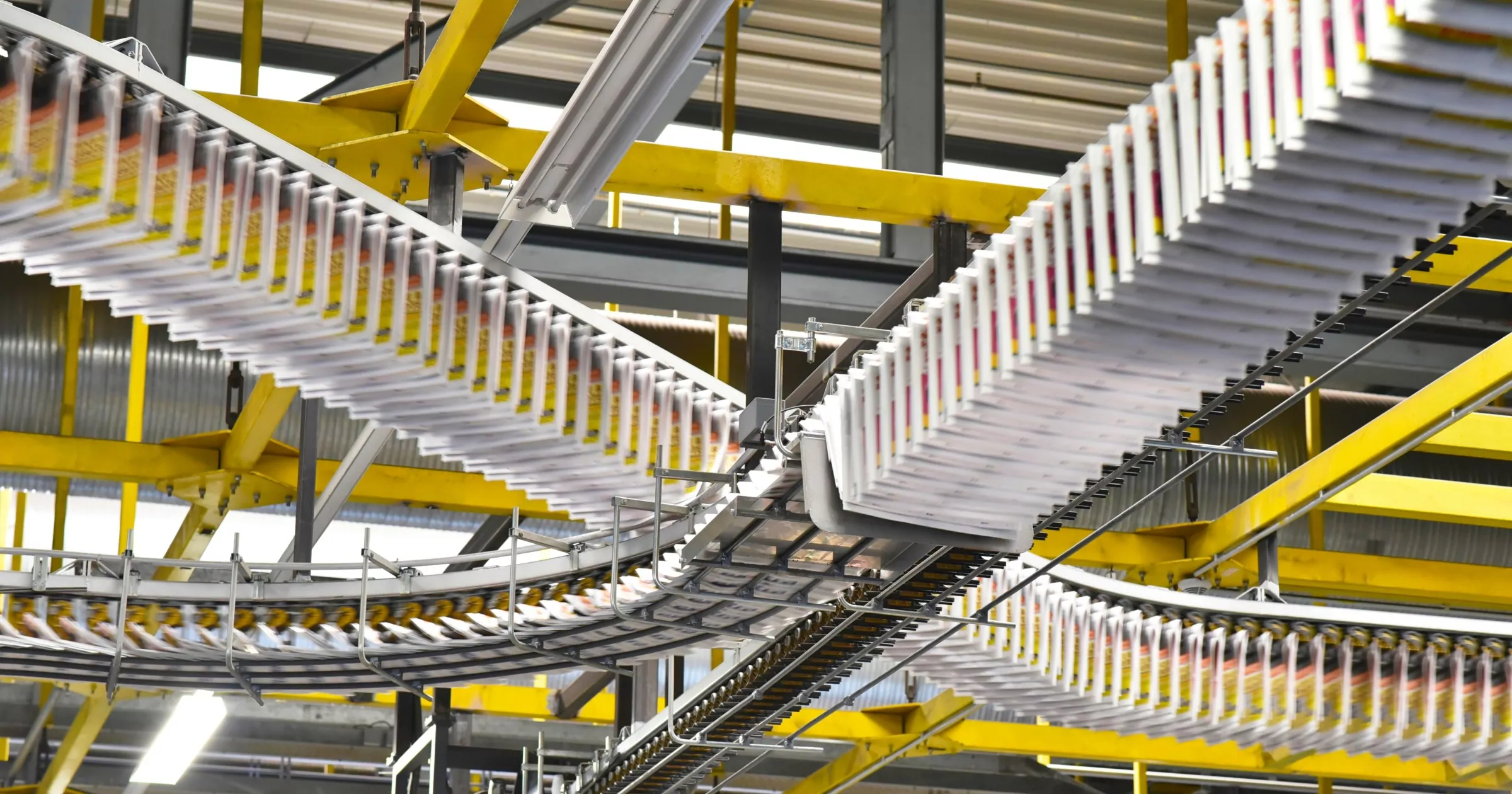
Manufacturing is in an inevitable position. We face cost pressures, supply chain volatility, and a constant barrage of disruptive technologies such as 3D printing and IoT. The industry must continually optimize processes, improve efficiency, and improve overall equipment efficiency.
At the same time, there is a huge sustainability and energy transition wave. Manufacturers are being asked to reduce their carbon emissions, adopt circular economy practices, and become more environmentally friendly overall.
And manufacturers face constant pressure to innovate while ensuring reliability and safety. Inaccurate AI predictions may be a minor problem in a marketing campaign, but inaccurate AI predictions in manufacturing can be fatal.
Technology and innovation are nothing new to manufacturers, but the biggest problem is that what works well in theory often fails in practice. For example, as a manufacturer, we create a knowledge base, but no one will be able to find anything unless they spend time searching and exploring the content. Or, you create a data lake that quickly degenerates into a data swamp. Or, you keep adding applications, so your technical debt continues to grow. However, the application cannot be modernized because logic developed over many years is hidden there.
The solution lies in generative AI
Let’s take a look at some of the features or use cases that are of most interest.
1. Summary
Summary remains a key use case for generative AI (gen AI) technology. Gen AI combined with search and multi-modal interaction makes it a great assistant. Manufacturers use summaries in a variety of ways.
They can use it to design better ways for operators to quickly and effectively retrieve the right information from vast repositories of operations manuals, SOPs, logbooks, past incidents, and more. This allows employees to focus more on their work and get their work done without unnecessary delays.
IBM® has a generation of manufacturing-focused AI accelerators for this purpose. Additionally, these accelerators are pre-integrated with a variety of cloud AI services and recommend the most appropriate large language models (LLMs) for your domain.
Summary is helpful even in harsh operating environments. When a machine or equipment fails, maintenance engineers can use Gen AI to quickly diagnose the problem based on maintenance manuals and analysis of process parameters.
2. Understand data in context
Data systems often pose major challenges for manufacturing companies. They are often disparate, siled, and multimodal. Various initiatives to create knowledge graphs for these systems have been only partially successful due to the depth of legacy knowledge, incomplete documentation, and decades of technical debt incurred.
IBM has developed an AI-based Knowledge Discovery system that uses generative AI to unlock new insights and accelerate data-driven decision-making with contextual industrial data. IBM has also developed accelerators for context-aware feature engineering in the industrial domain. This provides real-time visibility into process health (normal/abnormal), mitigates frequent process disruptions, and detects and predicts golden batches.
IBM has built a workforce advisor that uses summary and contextual data understanding through intent detection and multimodal interaction. Operators and plant engineers can use it to quickly focus on problem areas. Users can ask questions via voice, text, or pointing, and Gen AI Advisor processes and responds while recognizing the situation. This allows root cause analysis to be performed faster, saving time and effort, thus reducing the cognitive burden on users.
3. Coding support
Gen AI also helps with coding, including code documentation, code modernization, and code development. Consider the Water Corporation use case as an example of how Gen AI can help modernize IT. Water Corporation adopted Watson Code Assistant, powered by IBM’s Gen AI capabilities, to help it transition to a cloud-based SAP infrastructure.
The tool uses AI-generated recommendations based on natural language input to accelerate code development and significantly reduce deployment time and manual effort. Using Watson Code Assistant, Water Corporation reduced development effort and associated costs by 30% while maintaining code quality and transparency.
4. Asset management
Gen AI has the power to transform wealth management.
Generative AI can create underlying models for assets. When you need to forecast multiple KPIs in the same process or have multiple similar assets. It’s a good idea to develop one base model of your assets and fine-tune it multiple times.
Gen AI can also be trained for predictive maintenance. Baseline models are very useful when failure data is lacking. Existing AI models require many labels to provide reasonable accuracy. However, in our baseline model, we can pre-train the model without labels and fine-tune it with limited labels.
Generative AI can also provide technician support and training. Manufacturers can use Gen AI technology to create training simulators for operators and technicians. Additionally, during the repair process, Gen AI technology can provide guidance and create the best repair procedure.
Building new digital capabilities with generative AI
IBM believes that the agility, flexibility and scalability offered by generative AI technologies will significantly accelerate digitalization initiatives in the manufacturing industry.
Generative AI empowers companies at the strategic core of their business. Within two years, the underlying model will support approximately one-third of AI in enterprise environments.
IBM’s initial work applying foundational models shows time to value is up to 70% faster than traditional AI approaches. Generative AI can help manufacturing companies realize the value of their investments by leveraging other AI and analytics technologies.
Building new digital capabilities with generative AI
Was this article helpful?
yesno