Example of using AO Core libraries for self-optimization – Miscellaneous – March 5, 2024
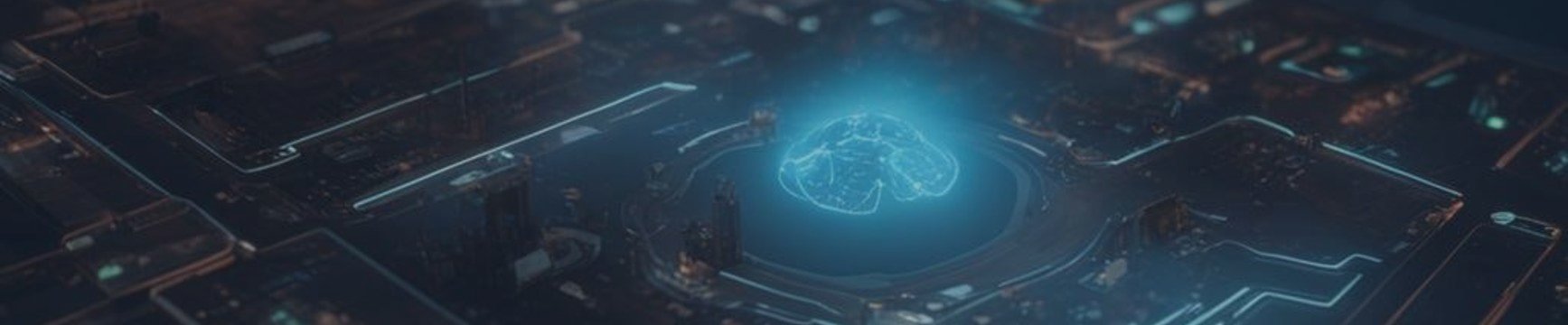
AO core
To ensure self-optimization of Advisor to implement the required functionality, the scheme presented in Figure 1 is used.
In the “historical” timeline, Advisor is positioned at the “as-now” point where optimization decisions are made. The “EA” advisor calls a “manager function” that manages the optimization process, with the advisor passing optimization settings and “optimization parameters” to this function.
The manager then requests a set of parameters from the optimization algorithm “Optimization ALGO” or “AO”. These will be referred to as “sets” from now on. After that, the manager passes the set to the virtual trading strategy “EA Virt”, which is completely similar to the real strategy of executing the trading operation “EA”.
“EA Virt” engages in virtual trading from “past” times in history to “now” times. The administrator starts the execution of “EA Virt” the number of times specified by the population size in “Optimization Parameters”. “EA Virt” then returns the past execution results in “ff result” format.
“ff result” refers to the fitness function result or optimization criterion, which can be varied at the user’s discretion. For example, this could be a balance, profit factor, mathematical expectation or complex criterion, integral or gross differential measured at various points in the “historical” timeline. Therefore, the fitness function result or “ff result” is what users consider to be an important indicator of the quality of their trading strategy.
Afterwards, a specific set of evaluations, the “ff results”, are passed by the manager to the optimization algorithm.
Once the stopping condition is met, the manager transfers the best set to the trading advisor “EA”, after which the advisor continues operations (trading) using the updated parameters from the “now point” point to the reoptimization point “reoptimiz”. Reoptimization is performed up to the specified depth of history.
The reoptimization point can be selected based on a variety of considerations. It may be a fixed number of historical bars, as in the example provided below, or it may be a specific condition, such as a trading indicator decreasing to a critical level.
Figure 1.
According to the way the optimization algorithm ‘Optimization ALGO’ works, it can be viewed as a ‘black box’ (in fact, everything outside is also a ‘black box’) that operates autonomously, regardless of the specific trading strategy. , managers and virtual strategies. The manager requests a set from the optimization algorithm and sends back an evaluation of this set, which the optimization algorithm uses to determine the next set. This cycle continues until you find the best set of parameters that meet your requirements. Therefore, the optimization algorithm finds optimal parameters that specifically meet the user’s requirements defined by the fitness function of “EA Virt”.
Indicator virtualization
Running the Advisor on historical data requires creating a virtual copy of your trading strategy that will execute the same trading operations as you would when working on a trading account. If you are not using indicators, virtualizing logical conditions within Advisor is relatively simple. Simply describe the logical course of action based on the point in the price series. Using indicators requires more complex operations, but in most cases trading strategies rely on the use of various indicators.
The problem arises when searching for optimal indicator parameters, because we need to create indicator handles with the current set in a given iteration. These handles should be deleted after running historical data. Otherwise, your computer’s RAM may fill up quickly, especially if you have a large set of potential parameters. This would not be a problem if you performed this procedure on a symbol chart, but the tester does not allow handle deletion.
To solve this problem, we need to “virtualize” the indicator calculation within the execution advisor to avoid using handles. Let’s take the stochastic indicator as an example.
The calculation part of each indicator contains a standard function called “OnCalculate”. This function should be renamed to “Calculate”, for example, and should remain largely unchanged.
Indicators must be organized into classes (structures work too). Let’s name it “C_Stochastic”. In the class declaration, the main indicator buffer must be defined as a public field (additional calculation buffers can be private) and an initialization function “Init” must be declared, to which the indicator parameters must be passed.
class C_iStochastic public: void Init (const int InpKPeriod, const int InpDPeriod, const int InpSlowing) inpKPeriod = InpKPeriod; inpDPeriod = InpDPeriod; inpSlowing = InpSlowing; public: int Calculate (const int rates_total, const int prev_calculated, const double &high (), const double &low (), const double &close ()); public: double ExtMainBuffer (); public: double ExtSignalBuffer (); private: double ExtHighesBuffer (); private: double ExtLowesBuffer (); private: int inpKPeriod; private: int inpDPeriod; private: int inpSlowing; ;
Therefore, in the “Calculate” method the actual calculation of the indicators is carried out. Calculating indicators is no different from those in standard terminal settings. The only difference is the size allocation and initialization of the indicator buffer.
This is a very simple example to understand the principles of metric virtualization. Calculations are performed over the entire period specified in the indicator parameters.
int C_iStochastic::Calculate (const int rates_total, const int prev_calculated, const double &high (), const double &low (), const double &close ()) if (rates_total <= inpKPeriod + inpDPeriod + inpSlowing) return (0); ArrayResize (ExtHighesBuffer, rates_total); ArrayResize (ExtLowesBuffer, rates_total); ArrayResize (ExtMainBuffer, rates_total); ArrayResize (ExtSignalBuffer, rates_total); ArrayInitialize (ExtHighesBuffer, 0.0); ArrayInitialize (ExtLowesBuffer, 0.0); ArrayInitialize (ExtMainBuffer, 0.0); ArrayInitialize (ExtSignalBuffer, 0.0); int i, k, start; start = inpKPeriod - 1; if (start + 1 < prev_calculated) start = prev_calculated - 2; Print ("start ", start); else for (i = 0; i < start; i++) ExtLowesBuffer (i) = 0.0; ExtHighesBuffer (i) = 0.0; for (i = start; i < rates_total && !IsStopped (); i++) double dmin = 1000000.0; double dmax = -1000000.0; for (k = i - inpKPeriod + 1; k <= i; k++) if (dmin > low (k)) dmin = low (k); if (dmax < high (k)) dmax = high (k); ExtLowesBuffer (i) = dmin; ExtHighesBuffer (i) = dmax; start = inpKPeriod - 1 + inpSlowing - 1; if (start + 1 < prev_calculated) start = prev_calculated - 2; else for (i = 0; i < start; i++) ExtMainBuffer (i) = 0.0; for (i = start; i < rates_total && !IsStopped (); i++) double sum_low = 0.0; double sum_high = 0.0; for (k = (i - inpSlowing + 1); k <= i; k++) sum_low += (close (k) - ExtLowesBuffer (k)); sum_high += (ExtHighesBuffer (k) - ExtLowesBuffer (k)); if (sum_high == 0.0) ExtMainBuffer (i) = 100.0; else ExtMainBuffer (i) = sum_low / sum_high * 100; start = inpDPeriod - 1; if (start + 1 < prev_calculated) start = prev_calculated - 2; else for (i = 0; i < start; i++) ExtSignalBuffer (i) = 0.0; for (i = start; i < rates_total && !IsStopped (); i++) double sum = 0.0; for (k = 0; k < inpDPeriod; k++) sum += ExtMainBuffer (i - k); ExtSignalBuffer (i) = sum / inpDPeriod; return (rates_total);
Strategy Virtualization
After discussing the virtualization of metrics within Advisor, we now move on to considering the virtualization of strategies. At the beginning of the Advisor’s code, we declare library imports, including files from the standard trading library and pseudo-stochastic files.
These are the Advisor’s “input” parameters: Among them, we highlight “InpKPeriod_P” and “InpUpperLevel_P”. These parameters should be optimized to represent the period and corresponding level of the “stochastic” indicator.
input string InpKPeriod_P = "18|9|3|24"; input string InpUpperLevel_P = "96|88|2|98";
It’s also worth noting that the parameter is declared as a string type. These parameters are complex and include default values, starting optimization values, phase, and final optimization values.
The advisor initialization within the “OnInit” function sets the size of the parameter array depending on the number of parameters to be optimized. “Set” – parameter set, “Range_Min” – minimum parameter value (starting value), “Range_Step” – parameter step, “Range_Max” – maximum parameter value. Extracts the corresponding values from a string parameter and assigns them to an array.
#import "\\Market\\AO Core.ex5" bool Init (int colonySize, double &range_min (), double &range_max (), double &range_step ()); void Preparation (); void GetVariantCalc (double &variant (), int pos); void SetFitness (double value, int pos); void Revision (); void GetVariant (double &variant (), int pos); double GetFitness (int pos); #import #include <Trade\Trade.mqh>; #include "cStochastic.mqh" input group "==== GENERAL ===="; sinput long InpMagicNumber = 132516; sinput double InpLotSize = 0.01; input group "==== Trading ===="; input int InpStopLoss = 1450; input int InpTakeProfit = 1200; input group "==== Stochastic ==|value|start|step|end|=="; input string InpKPeriod_P = "18|9|3|24"; input string InpUpperLevel_P = "96|88|2|98"; input group "====Self-optimization===="; sinput bool SelfOptimization = true; sinput int InpBarsOptimize = 18000; sinput int InpBarsReOptimize = 1440; sinput int InpPopSize = 50; sinput int NumberFFlaunches = 10000; sinput int Spread = 10; MqlTick Tick; CTrade Trade; C_iStochastic IStoch; double Set (); double Range_Min (); double Range_Step (); double Range_Max (); double TickSize = 0.0; /—————————————————————————————————————————————————————————————————————————————— int OnInit () TickSize = SymbolInfoDouble (_Symbol, SYMBOL_TRADE_TICK_SIZE); ArrayResize (Set, 2); ArrayResize (Range_Min, 2); ArrayResize (Range_Step, 2); ArrayResize (Range_Max, 2); string result (); if (StringSplit (InpKPeriod_P, StringGetCharacter ("
We also insert a block in the advisor code within the “OnTick” function that calls our own optimization. The “Optimize” function acts as the “manager” in the diagram in Figure 1 and starts the optimization process. If external variables requiring optimization are to be used, utilize the values in the “Set” array.
void OnTick () if (!IsNewBar ()) return; if (SelfOptimization) static datetime LastOptimizeTime = 0; datetime timeNow = iTime (_Symbol, PERIOD_CURRENT, 0); datetime timeReop = iTime (_Symbol, PERIOD_CURRENT, InpBarsReOptimize); if (LastOptimizeTime <= timeReop) LastOptimizeTime = timeNow; Print ("-------------------Start of optimization----------------------"); Print ("Old set:"); ArrayPrint (Set); Optimize (Set, Range_Min, Range_Step, Range_Max, InpBarsOptimize, InpPopSize, NumberFFlaunches, Spread * SymbolInfoDouble (_Symbol, SYMBOL_TRADE_TICK_SIZE)); Print ("New set:"); ArrayPrint (Set); IStoch.Init ((int)Set (0), 1, 3); if (!SymbolInfoTick (_Symbol, Tick)) Print ("Failed to get current symbol tick"); return; MqlRates rates (); int dataCount = CopyRates (_Symbol, PERIOD_CURRENT, 0, (int)Set (0) + 1 + 3 + 1, rates); if (dataCount == -1) Print ("Data get error"); return; double hi (); double lo (); double cl (); ArrayResize (hi, dataCount); ArrayResize (lo, dataCount); ArrayResize (cl, dataCount); for (int i = 0; i < dataCount; i++) hi (i) = rates (i).high; lo (i) = rates (i).low; cl (i) = rates (i).close; int calc = IStoch.Calculate (dataCount, 0, hi, lo, cl); if (calc <= 0) return; double buff0 = IStoch.ExtMainBuffer (ArraySize (IStoch.ExtMainBuffer) - 2); double buff1 = IStoch.ExtMainBuffer (ArraySize (IStoch.ExtMainBuffer) - 3); int cntBuy, cntSell; if (!CountOpenPositions (cntBuy, cntSell)) Print ("Failed to count open positions"); return; if (cntBuy == 0 && buff1 <= (100 - (int)Set (1)) && buff0 > (100 - (int)Set (1))) ClosePositions (2); double sl = NP (Tick.bid - InpStopLoss * TickSize); double tp = NP (Tick.bid + InpTakeProfit * TickSize); Trade.PositionOpen (_Symbol, ORDER_TYPE_BUY, InpLotSize, Tick.ask, sl, tp, "Stochastic EA"); if (cntSell == 0 && buff1 >= (int)Set (1) && buff0 < (int)Set (1)) ClosePositions (1); double sl = NP (Tick.ask + InpStopLoss * TickSize); double tp = NP (Tick.ask - InpTakeProfit * TickSize); Trade.PositionOpen (_Symbol, ORDER_TYPE_SELL, InpLotSize, Tick.bid, sl, tp, "Stochastic EA");
Likewise, the “Optimize” function does the same thing you would typically see in an optimization algorithm test script from the series of articles on “Population Optimization Algorithms”.
1. Initialization of the optimization algorithm.
2.1. Population preparation.
2.2. Obtain a set of parameters from the optimization algorithm.
2.3. Calculate the fitness function using the passed parameters.
2.4. Update the best solution.
2.5. The best solution is obtained from the algorithm.
void Optimize (double &set (), double &range_min (), double &range_step (), double &range_max (), const int inpBarsOptimize, const int inpPopSize, const int numberFFlaunches, const double spread) double parametersSet (); ArrayResize(parametersSet, ArraySize(set)); int epochCount = numberFFlaunches / inpPopSize; Init(inpPopSize, range_min, range_max, range_step); for (int epochCNT = 1; epochCNT <= epochCount && !IsStopped (); epochCNT++) Preparation (); for (int set = 0; set < inpPopSize; set++) GetVariantCalc (parametersSet, set); SetFitness (VirtualStrategy (parametersSet, inpBarsOptimize, spread), set); Revision (); Print ("Fitness: ", GetFitness (0)); GetVariant (parametersSet, 0); ArrayCopy (set, parametersSet, 0, 0, WHOLE_ARRAY);
Additionally, the “VirtualStrategy” feature tests strategies against historical data (referred to as “EA Virt” in Figure 1). It takes the following values: parameter array “set”, number of bars for optimization “barsOptimize” and “spread”.
The data preparation step comes first. Historical data is loaded into the “rates” array. Then the arrays “hi”, “lo” and “cl” required for the probabilistic calculation are generated.
Next, the stochastic indicator is initialized and calculations are performed based on historical data. If the calculation fails, the function returns “-DBL_MAX” (the worst fitness function value).
Afterwards, the strategy is tested against historical data following the same logic as the leading advisor’s code. A “Transaction” object is created to store transactions. The loop iterates through historical data, where it checks the position opening and closing conditions for each bar based on the indicator value and the “upLevel” and “dnLevel” levels. When the conditions are met, the position is opened or closed.
After looping through the historical data, the function checks the number of transactions executed. If no transaction was made, the function returns “-DBL_MAX”. Otherwise it returns the final balance.
The return value of “VirtualStrategy” represents the fitness function value. In this case, it is the final balance in points (as mentioned earlier, the suitability function can be a balance, a profit factor, or any other metric that indicates the quality of the trading results over historical data).
It is important to note that your hypothetical strategy should closely align with your advisor’s strategy. In this example, trading is based on the opening price corresponding to the main advisor’s bar opening control. If the trading strategy logic operates on all ticks, users should ensure that tick data is available during virtual testing and adjust the “VirtualStrategy” function accordingly.
double VirtualStrategy (double &set (), int barsOptimize, double spread) MqlRates rates (); int dataCount = CopyRates(_Symbol, PERIOD_CURRENT, 0, barsOptimize + 1, rates); if (dataCount == -1) Print ("Data get error"); return -DBL_MAX; double hi (); double lo (); double cl (); ArrayResize (hi, dataCount); ArrayResize (lo, dataCount); ArrayResize (cl, dataCount); for (int i = 0; i < dataCount; i++) hi (i) = rates (i).high; lo (i) = rates (i).low; cl (i) = rates (i).close; C_iStochastic iStoch; iStoch.Init ((int)set (0), 1, 3); int calc = iStoch.Calculate (dataCount, 0, hi, lo, cl); if (calc <= 0) return -DBL_MAX; S_Deals deals; double iStMain0 = 0.0; double iStMain1 = 0.0; double upLevel = set (1); double dnLevel = 100.0 - set (1); double balance = 0.0; for (int i = 2; i < dataCount; i++) iStMain1 == 0.0) continue; if (iStMain1 <= dnLevel && dnLevel < iStMain0) deals.ClosPos (-1, rates (i).open, spread); if (deals.GetBuys () == 0) deals.OpenPos (1, rates (i).open, spread); if (iStMain1 >= upLevel && upLevel > iStMain0) deals.ClosPos (1, rates (i).open, spread); if (deals.GetSels () == 0) deals.OpenPos (-1, rates (i).open, spread); if (deals.histSelsCNT + deals.histBuysCNT <= 0) return -DBL_MAX; return deals.balance;