Deep Learning and Machine Learning: Key Differences
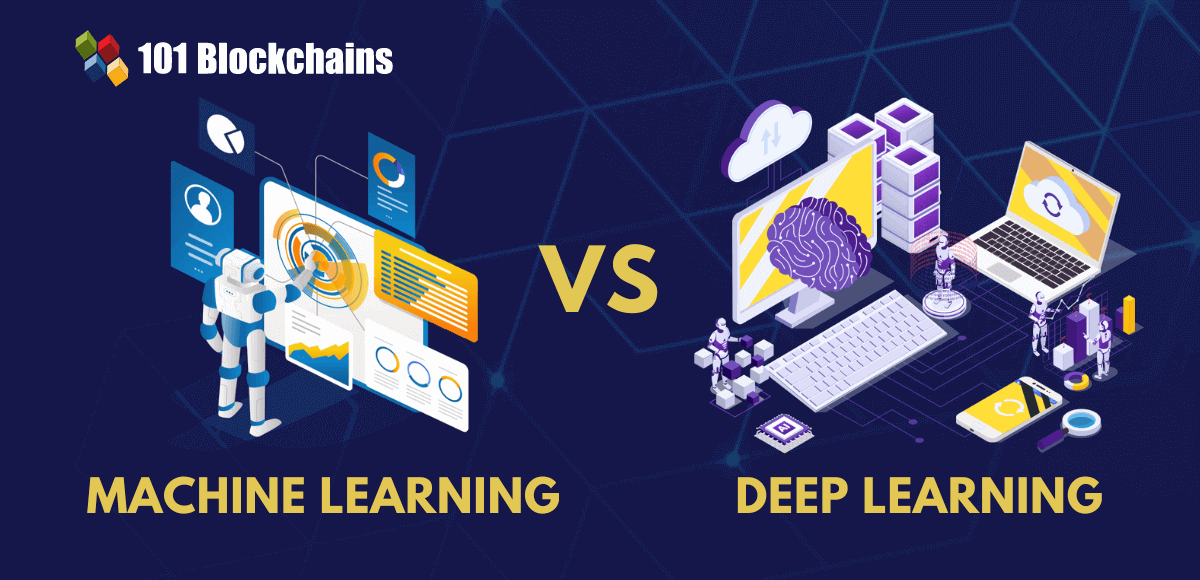
Digital technologies such as deep learning and machine learning have taken the world by storm. Although these technologies are in their infancy, they have shown tremendous potential. The emergence of these technological concepts benefits both individuals and businesses. Today, it has become essential to gain insight into the technologies that are revolutionizing our lives.
However, to understand these technologies at a comprehensive level, you need to understand not only their unique concepts but also the differences that exist between them. For anyone who wants to have a promising career in the highly competitive IT industry, it is important to know how concepts like DL and ML will impact the trajectory of AI. Before understanding the differences between deep learning and machine learning, it is important to have a clear knowledge of each technical concept.
What is machine learning?
Machine learning (ML) is a subdomain of artificial intelligence technology. ML primarily focuses on creating algorithms and statistical models that computer systems can learn. ML allows computers to make decisions or predictions without having to be explicitly programmed.
Therefore, machine learning involves training algorithms that assist in identifying relationships and patterns. Ultimately, these patterns help us make predictions about new data. Simply put, ML is a type of artificial intelligence that involves minimal human intervention.
Some of the key features of machine learning include:
- ML algorithms allow you to automate processes, helping you find patterns in your data.
- Machine learning technology can handle significant amounts of data, making it ideal for big data processing.
- Innovative technologies can help predict future events.
- ML algorithms can discover broad patterns in data that can help analyze new data that has not yet been explored.
Take the first step toward learning artificial intelligence with AI flashcards.
What is deep learning?
Deep learning is a part of machine learning. Analyze complex associations and patterns in data using multiple layers of neural networks. By imitating the human brain, it can perform a wide range of tasks, including voice recognition and natural language processing. One of the key characteristics of deep learning models is that they can be trained. Therefore, it can improve over time and the level of accuracy can improve as data is processed.
Some of the key features of deep learning include:
- Deep learning technology works by mimicking the human brain.
- The deep neural networks that make up DL have numerous layers that support models that learn hierarchical representations of data.
- DL’s characteristics of high power and flexibility make it suitable for a variety of tasks, such as speech and image recognition.
- Deep learning algorithms are very useful when there are many inputs and outputs.
To the average person, the terms artificial intelligence, machine learning, and deep learning may sound similar. The reality is that each of these concepts is different. But they are related. Now that you have a clear understanding of deep learning and machine learning concepts, it’s time to answer the following questions: What is the difference between deep learning and machine learning?
Do you want to learn the basics of AI applications in business? Enroll in the AI For Business course today
Differences between deep learning and machine learning
There are many differences between deep learning and machine learning. By identifying the uniqueness of a technology concept, you can identify its true potential.
Machine learning is a branch of artificial intelligence. Predictions are possible because statistical models and algorithms have been trained. This technology leverages historical data and training to build capabilities. The key thing to keep in mind is that ML relies on human intervention to feed and improve data.
Deep learning can be defined as a subset of machine learning techniques. A model that helps the algorithm determine whether its predictions are accurate. In deep learning, the role of neural networks is essential. Neural networks help software understand complex patterns, eliminating the need for human intervention.
The difference between machine learning and deep learning arises from the use of data representation. In the case of machine learning, the data representation used is quite different from that of deep learning. Machine learning uses structured data. This type of data is extremely valuable because it can be retrieved and analyzed in a simple and efficient way. ML techniques can be difficult to work with unstructured data. This is due to the fact that the training data set can have infinite variations.
The data representation used in deep learning is different because it involves neural networks. DL can handle structured as well as unstructured data. This technique can be used on a wide range of unstructured data, such as images, audio, text, as well as tables containing data.
Values arranged in a structure are called data points. Machine learning involves thousands of data points, while deep learning involves millions of data points. This feature shows that Machine Learning can work well even with relatively small databases. Deep learning, on the other hand, relies on huge amounts of data while performing its tasks. DL inherently requires large amounts of data to understand and perform tasks better than traditional ML algorithms.
The main difference that arises while exploring deep learning and machine learning is related to training time. Training time is basically the time taken by the model for the purpose of training on the data set. For ML algorithms, less time is required for training. Training time can take anywhere from a few seconds to several hours. But this is not the case with deep learning. Training times for deep learning neural networks can range from hours to weeks. However, it may be possible to adopt certain methods to reduce training time.
-
Efficiency and setup time
For machine learning, it may be possible to get the system up and running right away. However, just because a system is easy to build does not guarantee its efficiency. This may actually reduce the efficiency of the system. Conversely, setting up deep learning may seem time-consuming, but there is no compromise on the efficiency of the system. Once the system is up and running, limited or no intervention is required. It can produce results in an effective way. Moreover, the quality of the results is likely to improve over time.
The resources required for ML and DL systems can be quite different. It is possible to run machine learning on simple, standard computer systems. ML does not require large amounts of computational power, so it can be supported on standard computers. But the same cannot be said about deep learning.
Deep learning systems require powerful hardware and software resources to function properly. DL involves a wide range of tasks and requires robust systems to support them. Without adequate resources, the efficiency and functionality of DL can be compromised.
Do you want to understand the importance of ethics in AI, ethical frameworks, principles and challenges? Enroll in our Artificial Intelligence (AI) Ethics course now
The main difference between deep learning and machine learning has to do with the featurization process. In machine learning, it is important for users to accurately identify and generate features. This shows that the technology relies on users to perform its tasks and functions. However, deep learning technology does not require user intervention. DL can learn high-level features from the data itself. That’s not all! Deep learning can create new functions on its own without relying on human help or support.
The table below compares deep learning and machine learning.
<pre>Please include attribution to 101blockchains.com with this graphic. <a href="https://101blockchains.com/blockchain-infographics/"> <img src="https://101blockchains.com/wp-content/uploads/2024/05/Deep-Learning-vs-Machine-Learning.png" alt="deep learning vs machine learning="0' /> </a>
The future of ML and DL
In this technology-driven era, the future of machine learning and deep learning is full of possibility and potential. The unique features and capabilities of these technologies increase their ability to perform a wide range of tasks and operations. The future of ML and DL is very bright as AI technology reaches new levels.
If you want to pursue a career in the dynamic IT domain, expanding your knowledge of AI, ML, and DL is a must. Today, these technologies are catalyzing and influencing the trajectory of the IT industry. The advent of technologies such as machine learning and deep learning has created new job opportunities. If you are passionate about establishing yourself as a competent IT professional, you need to hone your skills related to ML and DL.
Unleash the full potential of generative AI in your business use cases and identify new ways to become an expert in generative AI technologies with the Generative AI Technology Path.
conclusion
In the artificial intelligence environment, technologies such as machine learning and deep learning play a key role. The main differences between ML and DL have been presented. Technology gives us a glimpse into the power of modern technology. There are many differences between deep learning and machine learning regarding basic concepts, data representation, use of data points, training time, efficiency, time resource requirements, and setting up the featurization process.
Today, both machine learning and deep learning are innovative technologies. The emergence of these technologies has brought tremendous value. The future of these technological concepts is full of high potential. These modern technologies have brought new opportunities and possibilities to the broader IT industry.