Interesting AI efficiency: Mixing small models outperforms larger ones.
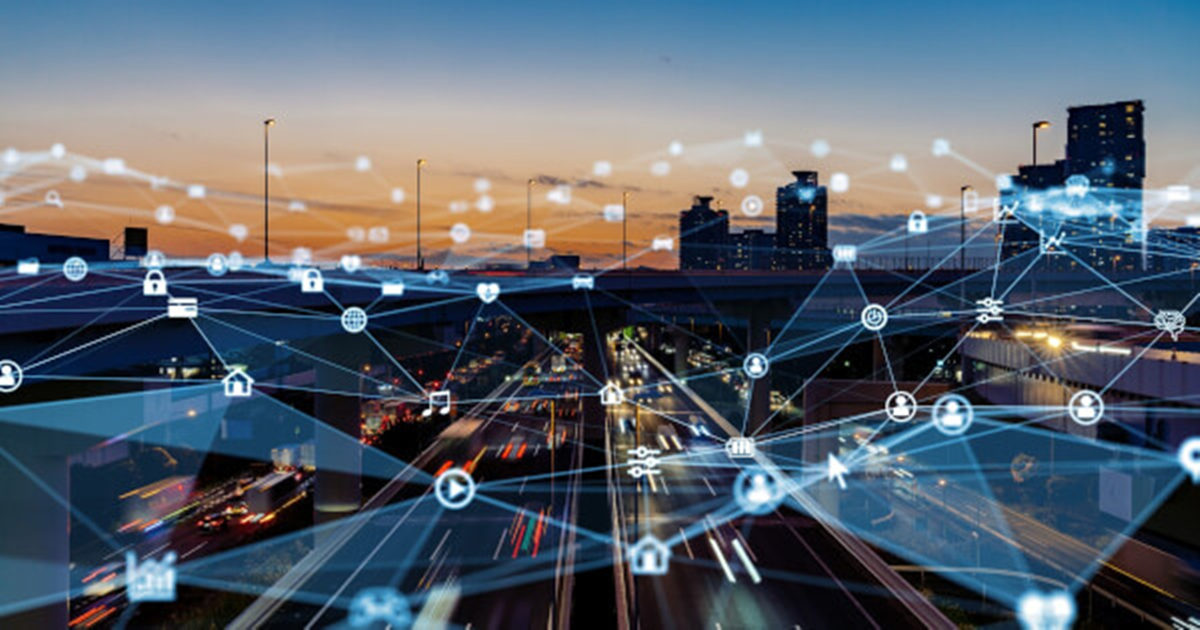
In recent years, the field of conversational AI has been heavily influenced by models such as ChatGPT, which feature a wide range of parameter sizes. However, this approach places significant demands on computing resources and memory. Now, a study has introduced a new concept that mixes multiple small AI models to achieve or exceed the performance of larger models. This approach, called “blending,” integrates multiple chat AIs to provide an effective solution to the computational problem of large-scale models.
A 30-day study conducted with a large user base on the Chai research platform shows that mixing certain small models can potentially match or surpass the capabilities of much larger models such as ChatGPT. For example, integrating just three models with 6B/13B parameters can match or even surpass the performance metrics of a much larger model, such as ChatGPT with 175B+ parameters.
The increasing reliance on pre-trained large language models (LLMs) in a variety of applications, especially chat AI, has led to a surge in the development of models with numerous parameters. However, these large-scale models require specialized infrastructure and have significant inference overhead, limiting their accessibility. On the other hand, a hybrid approach provides a more efficient alternative without compromising conversation quality.
The effectiveness of blended AI is evident in user engagement and retention. In a large-scale A/B test on the CHAI platform, a Blended ensemble of three 6-13B parameter LLMs outperformed OpenAI’s 175B+ parameter ChatGPT, achieving significantly higher user retention and engagement. This suggests that users find hybrid chat AI more engaging, fun, and useful, while requiring only a fraction of the inference cost and memory overhead compared to larger models.
The methodology of this study involves an ensemble based on Bayesian statistical principles. Here, the probability of a particular response is conceptualized as the marginal expectation for all plausible chat AI parameters. Blended randomly selects the chat AI currently generating the response, allowing different chat AIs to implicitly influence the output. This combines the strengths of individual chat AI to enable more engaging and varied responses.
Breakthrough trends in AI and machine learning in 2024 highlight the move toward more practical, efficient, and customizable AI models. As AI becomes more integrated into business operations, there is increasing demand for models that meet specific requirements and provide improved privacy and security. This change is consistent with the core principles of the blended approach, which emphasizes efficiency, cost-effectiveness, and adaptability.
In conclusion, the blended approach represents an important step forward in AI development. Combining multiple smaller models provides an efficient and cost-effective solution that maintains and, in some cases, improves user engagement and retention compared to larger, more resource-intensive models. This approach not only addresses practical limitations of large-scale AI, but also opens up new possibilities for AI applications across a variety of sectors.
Image source: Shutterstock