Nvidia: Navigating Competition Challenges And Exploring Growth Prospects (NASDAQ:NVDA)
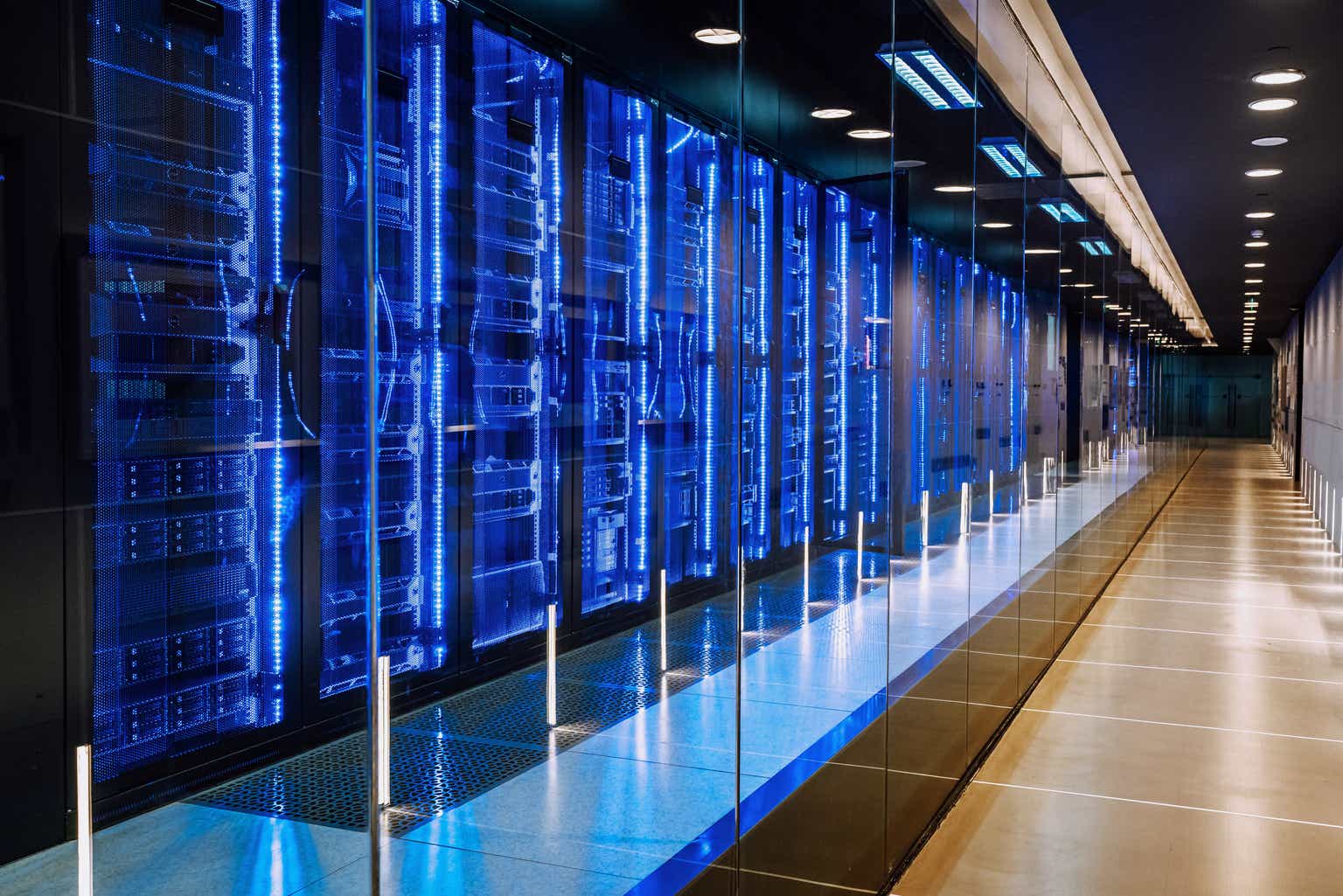
Nikada
Investment Thesis
Nvidia (NASDAQ:NVDA)(NEOE:NVDA:CA) has been the largest beneficiary of the surge in AI demand. They had the hardware and software in place and most importantly their systems were already in use for AI applications before Chat GPT’s viral explosion caused companies and countries globally to recognize the potential for AI. Do I feel the AI hype has been overblown, yes, but I also recognize the long-term potential this technology will have. My main critique is with “AI-Washing” companies that give exaggerated claims about their use of AI to garner investor and public attention. The FTC has directly warned against this practice because it could violate securities laws, and the SEC has started cracking down on false claims related to AI usage.
It will take several years for companies to develop fully formed products, but they will come eventually. That isn’t a problem for Nvidia though, and Nvidia’s surge in valuation is justified by the substantial revenue growth that unfolded over the last year and looks likely to continue.
Nvidia is well positioned for 2024 with demand for their products exceeding supply potentially lasting into 2025. What concerns me are the years to follow. Nvidia has a strong moat today, but there are many challengers, including Nvidia’s own customers that are working to replace them.
This article will primarily focus on challenges I see Nvidia facing in the years to come and how Nvidia’s expansion into premium software could act as a catalyst for future growth.
Q1 Earnings Highlights and 2024 Expectations
Nvidia set another record in Q1 with $26.04 billion in revenue (up 262% Y/Y) and $14.88 billion in net income (up 628% Y/Y) with a GAAP EPS of $5.98. They also announced a 10-for-1 stock split effective June 7th.
Most of their growth has been from the rapid build out of AI data center capacity. In their CFO commentary they said data center compute revenue was $19.4 billion (up 478% Y/Y and 29% Q/Q) and they made an additional $3.2 billion from networking. Cloud providers like Microsoft (MSFT)(MSFT:CA) and Amazon (AMZN)(AMZN:CA) were less of their total volume than I would have expected at “mid-40%” of data center revenue. This is a good sign for Nvidia because it implies more of their chips are going directly to customers who will use them than hyperscalers building out rentable servers.
During the earnings call, Jensen confirmed Blackwell “shipments will start shipping in Q2 and ramp in Q3, and customers should have data centers stood up in Q4”. Besides Blackwell, the H200 is also launching in Q2, and it was confirmed that the chips are in production. The H200 improves compute speed compared to the H100, and it adds the latest generation high bandwidth memory (HBM3e) leading to quicker data transfer speeds.
Looking ahead to Q2, management guided for revenues of $28.0 billion ($2B more than this quarter; 7.7% Q/Q growth). Gross margins are expected to be between 74.8% to 75.5%. On the earnings call they said that demand for their upcoming “H200 and Blackwell is well ahead of supply and we expect demand may exceed supply well into next year”. Nvidia looks to be in great shape this year, it will be interesting to see what Q3 and Q4 look like since their Q/Q growth rate appears to be slowing; not that 7.7% is bad.
Competition Challenges:
1. Intel and AMD – Late but Not Forgotten
Intel (INTC)(INTC:CA) and AMD (AMD)(AMD:CA) have been busy trying to catch up to Nvidia. Intel’s Gaudi 3 AI accelerator is 50% faster for training and 30% faster for inferencing than Nvidia’s H100. While AMD’s MI300X accelerator is able to keep up with Nvidia’s upcoming B100 (Blackwell). While the B100 beats the MI300X for smaller data types by roughly 30%, the MI300X wins for larger data types. Cost is also a critical element. The B100 is expected to cost between $30,000 and $35,000, while the MI300X cost $10,000 to $15,000. From a cost for performance perspective, AMD provides a more compelling chip.
Gaudi 3 Specs and Comparison to Nvidia (Intel)
Nvidia dominates the AI accelerator market. In Q1 FY25, Nvidia’s data center segment generated $22.5 billion in revenue and it’s fair to assume most of that was AI related. This is massive compared to the AI revenue generated by Intel or AMD. Intel expects to earn $500 million from Gaudi 3 sales this year because the chip is production limited, and AMD announced that their MI300X surpassed $1 billion in sales after 2 quarters of availability making it the fastest product ramp in their history. As Intel and AMD expand and improve their AI offering, they should capture more market share from Nvidia. Depending on market growth in the years ahead, that may mean shrinking sales for Nvidia if market expansion is less than market share loss.
2. Nvidia’s Customers are Creating In-House Accelerators
Nvidia’s largest customers are designing their own AI accelerators, and the main reason is cost. Amazon created their AWS Inferentia and Trainium AI accelerators to provide a lower cost and more efficient way to run and train AI programs. AWS was able to reduce costs 30-45% using their own hardware instances instead of GPU powered instances.
It’s not just Amazon making their own hardware either. Meta (META)(META:CA) has unveiled their in-house hardware, and so has Microsoft. Finally, Tesla’s (TSLA)(TSLA:CA) absolutely massive 25 die Dojo Training Tile was shown off during TSMC’s North American Technology Symposium.
Though these chips are less powerful than Nvidia’s and not as technologically advanced they are cheaper. At the end of the day cost rules and most applications don’t require the latest and greatest technology to operate. Whether an AI accelerator is used for inference or training also plays a large role in the demands placed on the chip (see point #5). Most of the in-house accelerators are designed for inference applications which will ultimately represent the largest AI market share. As the hyperscalers create their own chips their dependence on Nvidia will reduce. This will either reduce Nvidia’s margins because Nvidia will lower their price to keep sales up, or Nvidia’s revenue could fall.
3. Alternate Forms of Computing
Current AI accelerator hardware is based on the architectures used in GPUs. But there are alternate architectures to GPUs that could prove to be more efficient in a similar way that GPUs are more efficient than CPUs. There are three main competitors, analog, neuromorphic, and quantum. I’m going to skip quantum for now since it’s the farthest away.
Analog chips use physical properties to perform an action. Typically, these chips are found in sensors and other low-level interfaces between the physical and digital. Analog processors for AI applications are currently in the research stage. They are designed to store and process AI parameters using a physical method rather than digital processing. Matt Ferrell has a good YouTube video that gives an overview of how these systems operate. Analog chips present the possibility for reduced power consumption and faster processing speeds. IBM’s (IBM)(IBM:CA) protype analog processor can directly store and process the AI model parameters in memory leading to the aforementioned benefits.
Analog AI Chip (IBM Research)
Neuromorphic computing combines digital computing with structures designed to mimic neurons in a brain. These chips physically model neurons and their complex interactions while also providing discrete local processing. This reduces the need to move data (the most time-consuming step) while enabling highly parallel processing at far lower power usage. Intel’s Loihi 2 research chip has been used by multiple research institutions and it was recently announced that they will build the largest neuromorphic system in the world for Sandia National Laboratories.
4. Open-Source will Negate Nvidia’s Software Moat
Nvidia’s CUDA is a broad library of functions that makes it easier for programmers to add GPU acceleration to their programs. CUDA only works with Nvidia GPUs, and it is the true moat Nvidia possesses. As a result, developers looking to use competing hardware must create their own libraries.
Many large open-source projects are in the works to duplicate the capabilities of Nvidia’s libraries without placing hardware restrictions. This includes oneAPI, OpenVINO, and SYCL. Open source isn’t just for individuals who can’t afford Nvidia hardware. There is backing from large players like META, Microsoft, and Intel who are looking to break Nvidia’s moat by providing alternatives. Though there isn’t a fully featured CUDA alternative available today, the open-source community has shown its ability to replace and advance beyond the capabilities of proprietary software.
Growth Challenges:
5. Training vs Inference
AI models are created by training and used by inference. At the moment training is overrepresented in the total process demand. As AI programs become more prevalent inference will become the dominant workload. AWS expects inference will “comprise up to 90% of infrastructure spend for developing and running most machine learning applications”.
AI Training vs. Inference (Nvidia)
Training AI models requires substantial processing capacity, and this is where Nvidia’s GPU based AI accelerators excel. But inference is far less demanding. Traditional CPU designers like Apple (AAPL) (AAPL:CA) and Intel have integrated AI neural processors (NPUs) directly into their CPUs. They are integrating these capabilities to allow AI to run on device rather than requiring cloud execution. As AI processing becomes more integrated into our daily devices the need for cloud base inference will decrease. Additionally, chips designed for inference in the cloud don’t have the throughput requirements training chips have. This allows for less powerful but far cheaper chips to be used rather than Nvidia’s latest and greatest (see point 2).
6. Peak Data Center Growth
We are currently witnessing rapid growth in data centers globally and Nvidia has been capitalizing on the expansion. On Microsoft’s latest earnings call they discussed their upcoming $50 billion CAPEX spend this year alone and indicated that their spending will likely increase in the following years as well. They are spending heavily on cloud infrastructure because they want to be the cloud leader with sufficient capacity to support their clients. Not all new data center capacity is AI focused, but a large amount is. A research report by JLL, found that data centers are growing rapidly, from 10MW capacity in 2015 to over 100MW capacity being the new normal when building data centers. They also expect storage demand will grow at 18.5% CAGR, doubling the current global capacity by 2027. This rapid increase in data availability will be a critical component to the production of AI systems.
The high growth rate in AI demand poses two key risks. First is pull forward, second is slowing demand once AI finds its place within the product landscape. The rapid buildout of data centers over the coming years could result in a glut of capacity, especially if enterprise growth is slower than cloud providers expect. The other risk is the failure of businesses to create compelling AI products. Businesses are spending heavily on AI, and they will expect results. Eventually AI will find its place, but I doubt it will be the solution to all of the world’s problems; it is a tool with its own unique set of strengths and weaknesses. Both the pull forward of demand and failure to create valuable products could hurt Nvidia’s revenues in the future if customers decide they have sufficient processing capacity.
7. Small Models vs. Large Models
The most high-profile AI models such as ChatGPT are extremely large and have grown rapidly. ChatGPT-2 was 1.5 billion parameters, ChatGPT-3 was 175 billion parameters, and ChatGPT-4 is rumored to be 1.76 trillion parameters. They are powered by Nvidia accelerators and Nvidia has wisely used them as examples to attract more clients. But increasing size leads to increasing cost, and complexity to train and run the model.
Companies such as ServiceNow (NOW) have been focusing on creating smaller models that can be trained using customer specific proprietary data. Smaller models provide training and inference benefits because they have lower hardware demands and can more easily be tuned with proprietary data. I expect businesses will gravitate to smaller models due to the costs, time to market, and data demand. If smaller models become the norm, it will be easier for Nvidia’s competitors to take market share from Nvidia because customers don’t need the best.
Margin Challenges:
8. Mid-70% Gross Margins and 400% Annual Growth is Not Sustainable in the Long Run
In Q1 FY25 gross margins were 78.4%. In the prior year, Q1 FY24 gross margins were 64.6%. The increase in margin and revenue is directly attributable to growth in their data center segment. The chart below shows Nvidia’s gross profit and margins since 2020.
Nvidia Gross Margins and Profit (Nvidia Q1 FY25 Earnings Presentation)
High margins and explosive revenue growth (427% Y/Y in the data center segment) have been the cause of Nvidia’s share price growth over the past year. I see two potential risks, first Wall Street tends to be greedy and expect unrealistically high growth rates to persist, when growth stalls companies can drop rapidly (see Tesla, or every solar company). Secondly, Nvidia’s high margins will incentivize more competition, including Nvidia’s own customers. The increase in data center revenue is both a boon and potential bust for Nvidia. The customers most able to replace them are the customers driving the most revenue and growth. For Nvidia to maintain their market share in the long run they will need to be so far ahead of the competition that no one can catch up. However, it is more likely that Nvidia’s margins will get pushed down alongside decreasing market share. This could result in lower hardware revenue if volumes don’t increase enough to offset better pricing.
How Nvidia Can Thrive and Beat the Competition:
9. Keeping Their Hardware and Software Lead
This point is straightforward enough. Nvidia is currently in the lead with their CUDA platform providing foundational libraries needed to accelerate AI and a broad range of technical disciplines. Their hardware is also ahead of the competition (see point #1) for now, though the competition is heating up.
It is reasonable to expect Nvidia to maintain their leadership position for the foreseeable future because of their long history producing GPUs, current generational lead, and their emphasis on supporting AI, machine learning, big data, etc. with software before it was mainstream.
10. Software Revenue Could Lead the Next Wave of Growth
If you haven’t noticed I am uncertain about Nvidia’s ability to maintain their dominant hardware market share and I am concerned this will eventually hammer their stock price since most of their revenue comes from hardware. However, I see software as a largely untapped market for Nvidia. In the past the software Nvidia provided was for free to incentivize using their products. This has worked very well for them, and I expect their production of free software to persist going forward. But I also want to see Nvidia start to use the software they are making for themselves. Hardware is an important business, but the largest pile of money will be from software.
Nvidia does recognize the potential for software. The graph below shows their potential market opportunities. Hardware is presented as a $400 billion market, while software (just what is announced, who knows what is in R&D) could be worth $600 billion. Each software opportunity presents a new s-curve of potential growth, combined software makes an even greater opportunity than hardware. The following two points cover Nvidia’s steps into premium software.
$1 Trillion Long-Term Market Opportunity (Nvidia Q1 FY25 Earnings Presentation)
11. Nvidia’s AI Operating System
Nvidia AI Enterprise is a cloud-based container system designed to allow quick and efficient deployment of AI microservices. The system is like Kubernetes (which powers many cloud CPU applications) but it’s designed specifically for GPUs. Besides software, customers also receive technical support directly from Nvidia to help them maintain, upgrade, and troubleshoot problems.
Nvidia currently charges $4500 per GPU per year with an annual subscription. Nvidia has already achieved a $1 billion annual run rate from the system, and I expect that will increase as Nvidia’s installed hardware size grows. This is an important offering from Nvidia and will be a cornerstone of their premium software offerings. As long as Nvidia provides quality service they should see subscription revenues grow. SAAS companies receive high valuation multiples and reoccurring revenue will help maintain strong cash flow even if Nvidia is challenged on the hardware front.
12. Omniverse/Simulation Could be a Primary Revenue Driver if Monetized Right
Omniverse is a software package created by Nvidia to allow developers to integrate rendering and simulation capabilities in their applications. Omniverse is more powerful than traditional rendering engines such as Unreal because Omniverse is designed for integration and use with advanced simulation capabilities. Nvidia presents Digital Twins, Robotics Simulation, Synthetic Data, and Virtual Factory as main uses for this system. As an engineer, I can personally attest to the present usage of digital twins, and I expect they will become standard in the years to come.
A limiting factor for training AI is the large data requirements. Being able to create physically realistic virtual worlds will enable increased AI training and optimization without the risks associated with real world usage of an unfinished system. It should also enable faster training. For example, a robotics AI could learn how to assemble a product in a virtual world millions of times before being used in a real factory.
What’s not clear right now is Nvidia’s ability to monetize this system. It appears to be mostly aimed at selling hardware. Though this will generate some revenue, Nvidia has all the building blocks they need to branch out into other disciplines. For example, if Nvidia could create a high quality self-driving car AI they could benefit from hardware sales and substantial software sales. Development of self-driving software is in the works and Nvidia has partnered with multiple EV manufacturers—most in China—to use the platform.
Is Nvidia Worth Investing In?
I am giving Nvidia a hold rating for now. I expect Nvidia will perform well this year due to strong demand for their products. Additionally, highly followed companies tend to perform well following stock splits; even though nothing fundamentally changes post-split. This means Nvidia could see more near-term upside. However, I am a long-term buy and hold investor. Though Nvidia has performed spectacularly, and the run may continue, I see too many long-term headwinds that will start to be felt as competitors refine their offerings.
Nvidia has a forward P/E around 40, which is not bad given the growth they have had, but this assumes they can maintain 75% +/- margins. Unless Nvidia can rapidly grow their software sales we may be witnessing peak Nvidia. Peaking could be marked by an eventual drop in share price as competition takes market share and pressures margins. Alternatively, Nvidia could grow into their current market cap, the share price would remain roughly flat while growth goes toward lowering their P/E and making up for reduced margins. The only way I see Nvidia maintaining long-term growth is through an expansion in premium software suites.
Conclusion & Risks
Nvidia is a risky stock in my opinion. Their rapid growth has justified the parabolic move upward in their share price, but there are limits to growth, and I see the current narrative around AI to be very bubbly in a similar way to blockchain/crypto/NFTs a few years ago. I don’t expect Nvidia to crash, but I also see little upside to be had once momentum runs out.
Nvidia has an undeniable lead for AI workloads, but the competition is coming. Just because they are behind doesn’t mean they will always be behind. A large part of Nvidia’s strength is their software libraries. They have only been about 1 generation ahead of the competition when it comes to hardware. If one of Nvidia’s competitors—whether its Amazon, AMD, Intel, Meta, Microsoft, or someone else not yet on the radar —can make a chip that is 80% as good for 40% the cost and Nvidia’s software moat has been chipped away, things won’t end well for Nvidia.
However, if Nvidia can produce foundational software and support systems, like Nvidia AI Enterprise, which provide value to customers that open-source software cannot then Nvidia could maintain market share. Premium software is only a fraction of their revenue today, if Nvidia can enter this market then their growth story could continue. However, if they remain reliant on hardware growth Nvidia’s momentum will likely run out.