NVIDIA NeMo-Aligner enhances supervised fine-tuning with data-efficient knowledge distillation.
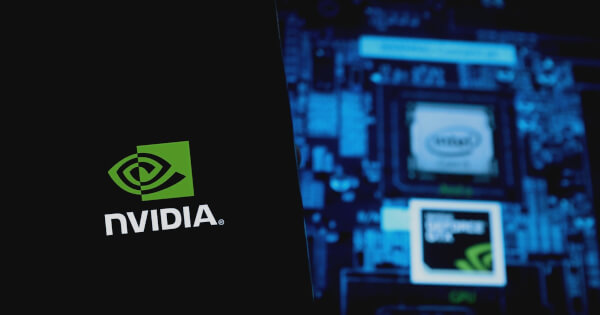
Peter Jang
December 18, 2024 09:40
NVIDIA NeMo-Aligner improves the performance and efficiency of neural models by introducing a data-efficient approach to knowledge distillation for supervised fine-tuning.
NVIDIA’s NeMo-Aligner has unveiled a new methodology to improve supervised fine-tuning (SFT) through data-efficient knowledge distillation. According to NVIDIA, this innovative approach allows knowledge to be transferred from a larger teacher model to a smaller student model, achieving similar accuracy while reducing data requirements.
Advances in Knowledge Distillation
Knowledge distillation is a technique that has been widely used in pre-training scenarios but is less explored in the context of supervised fine-tuning. NeMo-Aligner aims to bridge this gap by leveraging knowledge distillation during SFT to improve model accuracy and efficiency. This method achieves higher accuracy than standard SFT by utilizing only 70% of the training steps, as demonstrated in experiments.
Implementation and Benefits
NeMo-Aligner uses the KD-logit approach. Here, the student model is trained to match the teacher’s output logit. Known as “dark knowledge,” this technique understands the similarities and differences between classes to provide more informative gradient signals. This process includes preprocessing where the teacher model’s predictions are cached, and the student model is trained on these predictions, saving memory and reducing training time.
This approach saves GPU memory by significantly reducing the need to load teacher and student models simultaneously. Instead, only the top K logits of teachers are stored, optimizing memory usage while maintaining detailed information transfer.
empirical results
Experiments conducted using the Nemotron-4 15B student model and the fine-tuned Nemotron-4 340B teacher model show that the KD-fine-tuned model outperforms the vanilla SFT model on several benchmarks, including HumanEval, MBPP, and MATH. In particular, the KD fine-tuned model requires fewer training tokens and achieves good performance on 6 out of 7 evaluation metrics.
The KD approach also excels on the MMLU benchmark, which evaluates a wide range of language understanding tasks, outperforming baselines in both zero-shot and 5-shot settings.
conclusion
NVIDIA’s implementation of knowledge distillation in NeMo-Aligner demonstrates that this technology not only improves model performance in data-poor environments, but also effectively synergizes with synthetic data generation (SDG) technology. As a result, it provides a powerful tool for developers looking to maximize model efficiency and accuracy through supervised fine-tuning.
Image source: Shutterstock